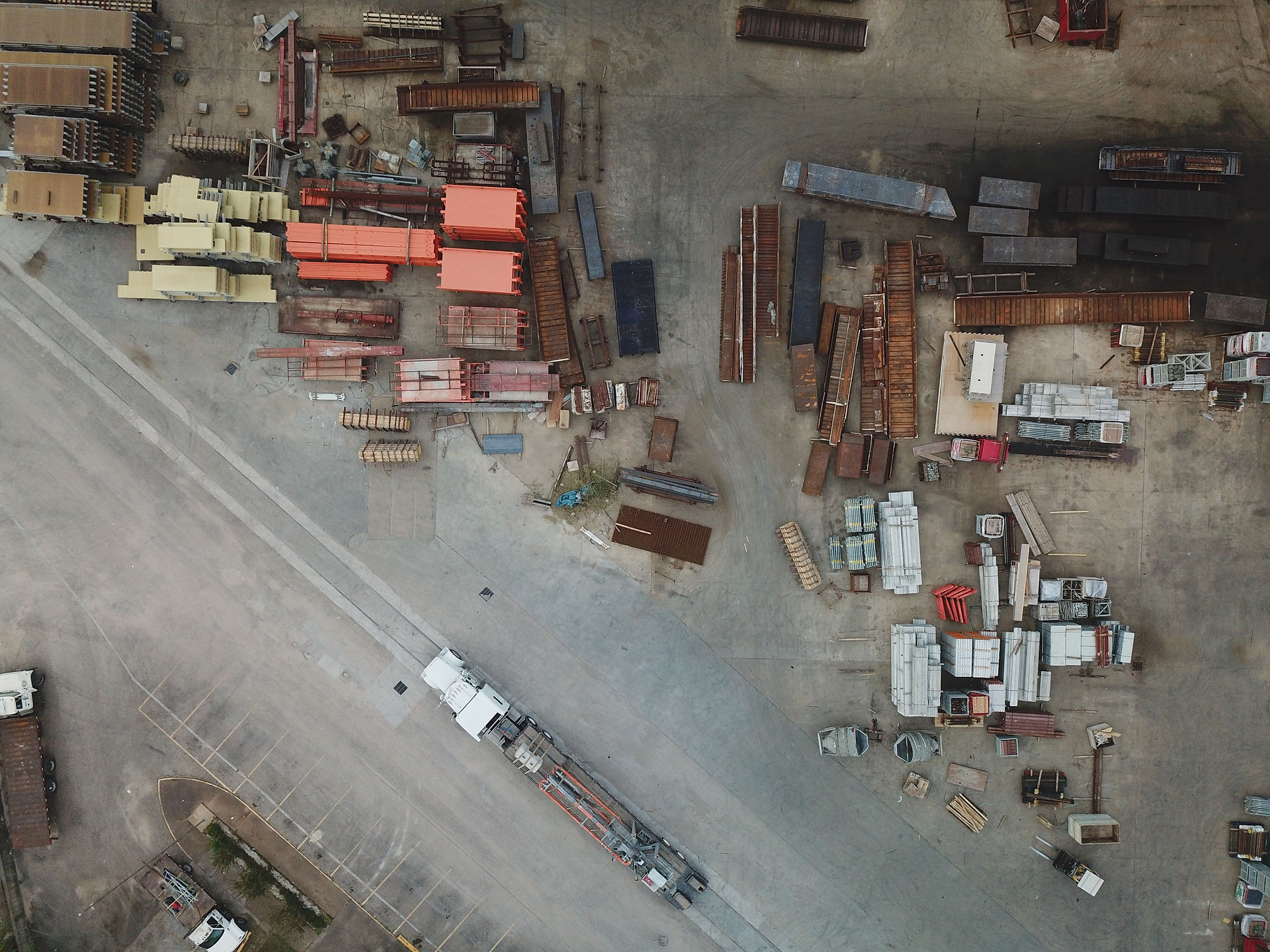
Corrosion is the biggest enemy of transmission pipelines. According to PHSMA, about 40% of pipeline damage and leakage is the direct result of corrosion, and other types of pipeline damage, such as excavation and natural force damage, are also highly associated with pipeline corrosion and can be easily triggered. Overall, soil corrosivity is the key and influences the degree of corrosion that happens on a pipeline.
​
In this section, the objective is to determine the soil corrosivity and assess the corrosion risks of pipelines by developing a continuous soil corrosivity index surface by using ArcMap and ArcGIS Pro in Harris County. Given the fact that soil corrosivity is influenced by a number of different soil properties at different levels, multi-criteria evaluation (MCE) was used as the technique to determine soil corrosivity in Harris County.
Corrosion is the biggest enemy of transmission pipelines. According to PHSMA, about 40% of pipeline damage and leakage is the direct result of corrosion, and other types of pipeline damage, such as excavation and natural force damage, are also highly associated with pipeline corrosion and can be easily triggered. Overall, soil corrosivity is the key and influences the degree of corrosion that happens on a pipeline.
​
In this section, the objective is to determine the soil corrosivity and assess the corrosion risks of pipelines by developing a continuous soil corrosivity index surface by using ArcMap and ArcGIS Pro in Harris County. Given the fact that soil corrosivity is influenced by a number of different soil properties at different levels, multi-criteria evaluation (MCE) was used as the technique to determine soil corrosivity in Harris County.
The lack of preparedness of local governments and energy companies led to this serious state-wide power outage and energy crisis. Therefore, to prevent similar series of disasters from happening, it is crucial to ensure and assess the resiliency and integrity of current transmission pipelines in the State of Texas.
In this project, the main objective is to investigate the driving factors of pipeline incidents (failures, accidents) of currently existing pipelines.
The lack of preparedness of local governments and energy companies led to this serious state-wide power outage and energy crisis. Therefore, to prevent similar series of disasters from happening, it is crucial to ensure and assess the resiliency and integrity of current transmission pipelines in the State of Texas.
In this project, the main objective is to investigate the driving factors of pipeline incidents (failures, accidents) of currently existing pipelines.
Section I: Human/Demographic Risk Assessment -
Geographically Weighted Regression Analysis
"One of the greatest challenges to safe pipeline operations is accidental damage to the pipe or its coating that is caused by someone inadvertently digging into a buried pipeline."
---- U.S. Pipeline & Hazardous Materials Safety Administration
Sometimes, any of the typical forms of excavation, such as new home construction, farming activities or infrastructure maintenance, can result in pipeline damages. As discussed previously, soil corrosivity sometimes does not directly cause a pipeline to break, but it weakens the resiliency of pipelines. The weakened pipelines could be easily broken or even destroyed by external forces. The external force, in most cases, is excavations that local residents proceeded with inadvertently.
​
In this section, the objective is to discover possible links between pipeline incident density and other human/demographic factors. Geographical Weighted Regression was applied to several important predictive variables to find the influences and local variations of these factors. To ensure the yielded results contain a sufficient amount of details, all input data were acquired at US Census Tracts (CTs) level.
Exploratory Regression
To investigate the most suitable set of explanatory variables, exploratory regression was first applied in ArcGIS Pro. In total, eleven variables were initially used as input in the exploratory regression.
Dependent Variable: Density of pipeline incidents. Calculated by applying Kernel Density to all recoded pipeline incidents in Harris County. Incident records from other surrounding counties were also included to enhance the results quality and eliminate modifiable areal unit problem.
Using the 11 input explanatory variables and the dependent variable listed above, a report was produced with summaries. The set of variables with the highest adjusted R-squared value and the lowest AICc (corrected Akaike Information Criteria) was then selected to be the most important explanatory variables since this set has the strongest relationships with the dependent variable. In this study, the exploratory regression yielded a set that consists of 4 explanatory variables, and these 4 variables were then used in the next part of the analysis.
Generalized Linear Regression (GLR)
GLR is a linear regression model that can either estimate predictions or model a dependent variable's relationships with a set of explanatory variables. GLR is a global regression, it does not take into account spatial variation. In this study, GLR was conducted in ArcGIS Pro to determine if there are any important explanatory variables missing in the model. The inputted set of variables were the ones identified in the Exploratory Regression mentioned above. From this analysis, the yielded residual values can reveal places where the model either overestimated or underestimated the observed values.
Geographically Weighted Regression (GWR)
Different than GLR, GWR is a regression model which considers spatial variations across a selected study area. That is, local variations and spatial relationships between the dependent variable and the explanatory variables can be modelled based on their spatial distribution by the GWR model. Here, GWR was applied via ArcGIS Pro to the identified explanatory variables for examining the spatial correlations between the explanatory variables and the pipeline incident density (the dependent variable) across the County of Harris.